This is a research paper from the Big Data and Smart Computing (BigComp) conference organized by IEEE in 2024.
Title: Dual Stream Fusion U-Net Transformers for 3D Medical Image Segmentation
Authors: Seungkyun Hong*, Sunghyun Ahn*, Youngwan Jo, and Sanghyun Park† [* co-first]
Status: Accepted (Oral Session)
Information: February 18-21, 2024, The Sukosol Hotel, Bangkok, Thailand
Conference Homepage: [BigComp 2024]
Academic Information Portal: [IEEE Xplore]
Github Code: [GitHub]
Access to paper: [PDF]
Abstract
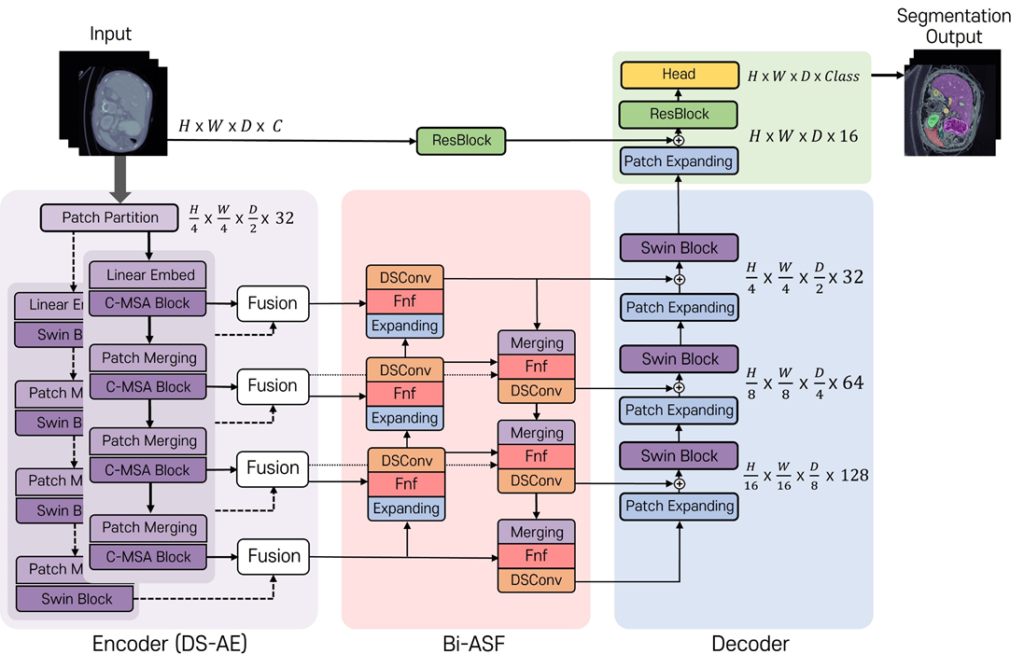
Medical image segmentation is a crucial ongoing issue in clinical applications for differentiating lesions and segmenting various organs to extract relevant features. Many recent studies have combined transformers, which enable global context modeling leveraging self-attention, with U-Nets to distinguish organs in complex volumetric medical images such as 3-dimensional(3D) Computed Tomography (CT) and Magnetic Resonance
Imaging (MRI) images. In this study, we propose a Dual Stream fusion U-NEt TRansformers (DS-UNETR) comprising a Dual Stream Attention Encoder (DS-AE) and Bidirectional All Scale Fusion (Bi-ASF) module. We designed the DS-AE that extracts both spatial and channel features in parallel streams to better understand the relation between channels. When transferring the extracted features from the DS-AE to the decoder, we used the Bi-ASF module to fuse all scale features. We achieved an average Dice similarity coefficient (Dice score) improvement of 0.97% and a 95% Hausdorff distance (HD95), indicating an improvement of 7.43% compared to that for a state-of-the-art model on the Synapse dataset. We also demonstrated the efficiency of our model by reducing the space and time complexity with a decrease of 80.73% in parameters and 78.86% in FLoating point
OPerationS (FLOPS). Our proposed model, DS-UNETR, shows superior performance and efficiency in terms of segmentation accuracy and model complexity (both space and time) compared to existing state-of-the-art models on the 3D medical image segmentation benchmark dataset. The approach of our proposed model can be effectively applied in various medical big data analysis applications.
Presentation