This is a research paper published in the IEEE Access journal in 2024.
Title: Making Anomalies More Anomalous: Video Anomaly Detection Using a Novel Generator and Destroyer
Authors: Seungkyun Hong*, Sunghyun Ahn*, Youngwan Jo, and Sanghyun Park† [* co-first]
Status: Published
Information: IEEE Access, year=2024, volume=12, pages=36712-36726
Academic Information Portal: [IEEE Xplore]
Github Code: [GitHub]
Access to paper: [PDF]
Abstract
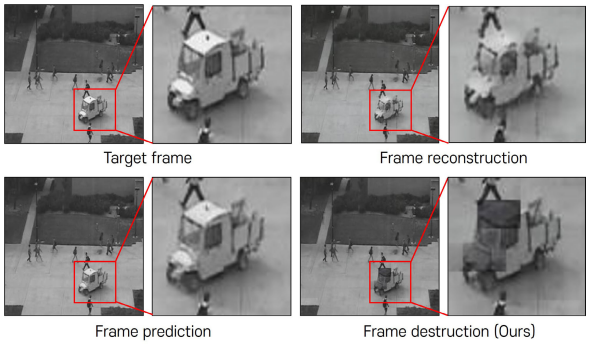
We propose a novel approach for video anomaly detection. Existing video anomaly detection methods train only on normal frames, with the expectation that the quality of the abnormal frames will decrease, and utilize the reconstruction error with the ground truth to detect anomalies. However, a challenge exists owing to the powerful generalization capability of deep neural networks, as they tend to proficiently generate abnormal frames. To address this issue, we introduce a novel method to make anomalies more anomalous by destroying abnormal areas in abnormal frames. Accordingly, we propose the frame-to-label and motion (F2LM) generator and Destroyer. The F2LM generator predicts a future frame by utilizing the label and motion information of the input frames, thereby degrading the quality of abnormal regions. The Destroyer destroys abnormal regions by transforming low-quality areas into zero vectors. Both models were trained individually, and during testing, the F2LM generator degraded the quality of abnormal regions, and the Destroyer subsequently destroyed these areas. Our proposed video anomaly detection method demonstrated superior performance compared to state-of-the-art models with three benchmark datasets (UCSD Ped2, CUHK Avenue, Shanghai Tech.). Our code and models are available online at https://github.com/SkiddieAhn/Paper-Making-Anomalies-More-Anomalous.
Presentation (KOR)